Forty thousand students in Connecticut are enrolled in failing schools. Eighty-seven percent of those students are Black or Hispanic, and ninety percent are low-income. 1 The historic Brown v. Board of Ed. case (1954) reaches its’ sixty first anniversary this year; it is difficult to realize many students are still segregated by socioeconomic class and race. Much of this segregation is evident in choice based schools, institutions geared toward providing educational opportunity to less advantaged students. 2 With an obligation to enroll 25-75% minority students, magnet schools are working to provide better education yet struggling to successfully integrate their classrooms. In this web essay, I aim to first understand how racial discrimination began in Connecticut schools and how it endures. Second, I will examine why classroom integration is important. Third, I will question whether choice based magnet schools, institutions initially created in an effort to provide greater opportunity to those who have less, are the best form of schooling to eradicate school-based segregation. Last, I will work to understand what the most effective solutions to racial segregation might be.
Why does racial segregation exist, and how can it be explained in schools in Hartford, CT?
Today, Connecticut is populated primarily by white, middle to upper class educated individuals. Some 81.6% of the population is white, with 11.3% Black and 14.7% Latino. For the years between 2009-2013, on average, 89.2% of residents across the state graduated from high school. Some 36.5% graduated from some form of secondary college education. 3 Even though Connecticut is white dominated, there are large pockets of minority individuals living throughout the state, especially in Bridgeport, Waterbury, New Haven, New Britain and East Hartford. Below is an interactive map application depicting the “racial breakdown of students since 1969, by district.” One can use the map to better understand school-based racial segregation throughout the state. 4
School-based segregation first began nationwide with Plessy v. Ferguson in 1896 and continued with Brown v. Board of Ed.’s (1954) poorly executed reparations. There are several ‘reasons’ for the ongoing segregation in Connecticut schools and in many others nationwide. Some of these explanations include proximity to schools, extracurricular activities, clubs, and religious temples, housing opportunities, white flight, gentrification and zoning. There are many causes; in this essay, I will speak of a few. In their work entitled “Smarter Charter,” Richard Kahlenberg and Haley Potter write, “Parents of all incomes were likely to value a strong reading and math curriculum. Learning good study habits and self-discipline also…the overall ranking of preferences was fairly consistent across income brackets”. 5. Even with the commonality of education goals, many still prefer enrolling their school-aged children in institutions of learning where in terms of race, ethnicity and culture, the student body is like their own. 6 People are motivated to live in communities with others like themselves as well. These areas of condensed poverty and wealth, and therefore further segregation, are depicted in the interactive map of Hartford below. 7
As demonstrated through self-selection practices, information neighborhood parents receive when enrolling their children in schools and the lottery system, school choice is yet another reason for much of the existing school-based segregation (131). 8 Many choice-based institutions target varied cultural and ethnic groups. 9 When they do this, certain racial groups have a stronger presence in the enrollment lotteries than others. The resulting grades and classes within schools become homogeneous. This has certainly been the case at Capital Preparatory Magnet School in Hartford, CT, which was “originally created to give students living in high poverty areas the chance to receive exceptional education in areas where it’s most needed.” 10
In 1980, Connecticut instituted the Racial Imbalance Law, requiring schools to report their racial make-up to the state. This information included proximity of home from schools and how many students require free or reduced price lunch. Schools were forced to communicate with their districts when the number of minority students was not aligned with that of the majority race students. 11 This law failed, and so has the state government in eradicating school-based segregation. The law did however opened up a dialogue that had not been previously discussed; that conversation is centered heavily on integration, its importance and how it can change the future of education today. 12
Understanding Integration and its importance in Connecticut schools:
In 1989, Elizabeth Horton Sheff began a crusade against Connecticut on behalf of minority students in the state, demonstrating Black and Latino schools in urban areas were less privileged than those of the white suburban schools. In 1996, district lines were deemed unconstitutional and within the following year, “An Act Enhancing Educational Choices and Opportunities” was passed; it encouraged racial integration through school choice. In 2008, a greater number of spots for students from all areas and racial backgrounds were to be made available in magnet schools. 13 14
As Sheff has helped to demonstrate, there are many benefits to racial integration in schools. Some include curtailing racism, providing a forum for mixed race friendships and increasing school-wide test scores in lesser performing schools. In her article entitled “What Will you Think of Me? Racial Integration, Peer Relationships and Achievement Among White Students and Students of Color,” Sabrina Zirkel writes, “Desegregated schools do produce more successful educational and professional outcomes for students of color. They do reduce prejudice and increase racial integration in the larger society. (58-59).” 15 Marguerite Spencer, a Professor at University of St. Thomas, finds children studying in integrated schools have “[a] higher level of parental involvement, higher graduation rates, complete more years of education, earn higher degrees and major in more varied disciplines, gain greater access to professional jobs and have higher incomes 16.
There are a surprising number of individuals disinterested and unwilling to send their children to integrated schools. In fact, the American Recovery and Reinvestment Act perpetuates school-based segregation, giving “upward of $70 billion to continue to reinforce patterns of racial and economic separation in American Schools.” 17 This is evidence racism is more alive than ever.
Are choice based schools the solution to limited diversity in schools?
Over the last decade, Connecticut state government has spent $1.4 billion on repairing those schools that are dilapidated and building new magnet schools. $140 million is spent on school upkeep each year. 18 One of the state’s goals has been to provide opportunities for 41% of Hartford’s minority students to enroll in integrated schools. Magnet schools initially seemed like the most likely approach. In the 2010-2011 school year, there were 2,722 magnet schools operating nationwide with 2,055,133 students enrolled. Fifty-four of those were in Connecticut. Today, there are seventy magnet schools in Connecticut serving 31,689 students, 70% of which are of minorities, mostly Black and Hispanic. 19
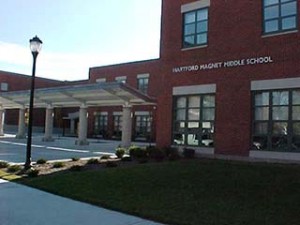
Magnet schools are often formed with a focused mission like STEM, Fine and Performing Arts, International Baccalaureate and Career and Technical Education. The curriculums are created in this manner in order to provide direction while also building family involvement. 20 21 These specialized programs were also created in an effort to attract white students to minority-majority schools, establishing a trend toward integration. Instead, as Boston University Professor Christine Rossell writes in her dissertation entitled “The Desegregation Efficiency of Magnet Schools,” “One possible explanation for why magnet schools [do] not have a more salutary effect on interracial exposure in the voluntary desegregation plans is that they may produce some white flight of their own.” (12) 22 “White flight” speaks to the racism many white people maintain and their resulting move to areas in which they make up the majority population (47) 23 Choice schools were created to provide opportunity to varied racial groups to converge to learn together, but classrooms in these institutions, especially in magnets, are still homogeneous. In order to understand why this is, an analysis of their assessment processes must be done.
Connecticut Magnet Schools are evaluated through a series of state designed questions each year. The first is “What characteristics define inter-district magnet schools and how do inter-district magnet schools differ from other public schools?” The second is, “what impact have inter-district magnet schools had on reducing the racial, ethnic, and economic isolation of Connecticut students?” The third is, “what impact have inter-district magnet schools had on reducing the racial, ethnic, and economic isolation of students within the magnet school itself?” The fourth is “How does the performance of inter-district magnet school students compare with that of public school students state-wide?” And the final two are “how consistent are students, parents, and their public school professional staff in their perception of the effectiveness of their magnet schools, and what characteristics do the highly successful magnet school share?” (3-5) 24. With these questions, magnet schools are clearly evaluated each year but their assesments are lacking. The questions themselves are fairly vague, keeping researchers from examining specific aspects of individual schools. Each inter-district magnet school does not maintain the same characteristics or the same students. Therefore, one school cannot be evaluated in the same exact way as another. Magnet schools are indeed effective in providing more opportunity than minority students would have previously had. They do not, however, provide the optimal education option of an integrated learning environment, especially when it comes to those students who are white or living with special needs. 25
What are the solutions to school-based segregation?
Since Brown v. Board of Ed., school-based segregation has become a larger problem than ever before. Much of this is due to the federal government’s limited implementation of relevant policy. No Child Left Behind was one reformation initiating efforts; testing alone though cannot close the achievement gap. Instead, as Gary Orfield suggests, districts need to first look at the factors outside schools that affect children in order to best understand why some schools have the demographics they do. Some of these include housing, day care, wages and health care. A parent’s ability to pay for housing in a middle to high income school district can dictate a child’s potential to enroll in a school with high levels of student success. Teachers must be provided with cultural sensitivity training so that they are equipped to instruct individuals of all backgrounds. Orfield also suggests universal pre-k, smaller class sizes, counseling and early grade reading classes will not only help to desegregate but will also help to close the achievement gap. 26 27 No one kind of institution can solve the conflict of racial segregation in schools. As Rod Dreher suggests, the US and Connecticut governments must make integration a priority, demanding a higher quality of teaching and a greater number of after school programs. Within the next ten years, white students will be in the minority. It is time individuals begin learning together with varied races now. 28
Conclusion:
With limited school-based integration, goals for institutions of learning to “promote an American identity, social cohesion and democratic leadership” are further out of reach than ever before. 29
Racial segregation can be an enormous impediment to learning, especially for low income minority students. Segregation encourages stereotypes, increases racism and widens the achievement gap by limiting access to quality education for Blacks and hispanics. Integration can help minorities more than it can the already racially privileged white students. It can assist in the efforts to raise scores on standardized tests, reduce violence, encourage parent involvement, create greater access to professional jobs and guarantee higher incomes. Integration and exposure to a variety of cultures and ethnicities can also help all students to eradicate racism and experience a life with greater perspective. 30
Choice-based institutions, like magnet schools, are meant to be building universal change. They are creating more opportunity for minority students, but not together with their majority peers. In an effort to build an integrated education system for the future, we must first understand how racial segregation began in Connecticut, how it endures, why integration is important and what the best solutions are. If choice-based schools have not been an effective form of segregation eradication, can we truly think of them as the future of our multi-cultural education system? I don’t think we can. The United States and Connecticut governments must make integration a top priority, creating a system of change that includes a higher standard for teaching and a greater availability of before and after school enrichment programs to foster equal access for all students, no matter what race, together.
Notes:
- 2014. Connecticut Education in Crisis: 40,000 Children Trapped in Failing Schools. connCAN. Retrieved May 11, 2015. (http://www.conncan.org/media-room/press-releases/2014-11-connecticut-education-in-crisis-40000-children-trapp)
- Frankenberg, E., Siegel-Hawley, G., & Wang, J. 2010. Choice without Equity: Charter School Segregation and the Need for Civil Rights Standards. Civil Rights Project/Proyecto Derechos Civiles. Retrieved April 30, 2015. (http://civilrightsproject.ucla.edu/research/k-12-education/integration-and-diversity/choice-without-equity-2009-report/)
- 2015. State and County QuickFacts: Connecticut. United States Census Bureau. Retrieved April 30, 2015.(http://quickfacts.census.gov/qfd/states/09000.html)
- (Thomas, Jacqueline Rabe. 2014. 60 Years after Brown vs. Board of Education: Still Separate in Connecticut. The CT Mirror. Retrieved May 1, 2015. (http://ctmirror.org/2014/05/16/60-years-after-brown-vs-board-of-education-still-separate-in-connecticut/).
- Kahlenberg, Richard D and Potter, Haley. 2014. A Smarter Charter: Finding what Works for Charter Schools and Public Education. New York, NY: Teachers College Press
- Kahlenberg, Richard D and Potter, Haley. 2014. A Smarter Charter: Finding what Works for Charter Schools and Public Education. New York, NY: Teachers College Press
- (Hartford, Connecticut (CT) Poverty Rate Data. City-Data.com. Advameg Inc. Retrieved May 10, 2015. (http://www.city-data.com/poverty/poverty-Hartford-Connecticut.html#mapOSM?)
- Orfield, Gary and Ee, Jongyeon. 2015. Connecticut School Integration: Moving Forward as the Northeast Retreats. UCLA The Civil Rights Project 5. Retrieved April 29, 2015. (http://civilrightsproject.ucla.edu/research/k-12-education/integration-and-diversity/connecticut-school-integration-moving-forward-as-the-northeast-retreats/orfield-ee-connecticut-school-integration-2015.pdf)
- Kahlenberg, Richard D and Potter, Haley. 2014. A Smarter Charter: Finding what Works for Charter Schools and Public Education. New York, NY: Teachers College Press
- 2011. Are Magnet Schools Perpetuating Segregation? The Huffington Post. Retrieved May 11, 2015. (http://www.huffingtonpost.com/2011/06/01/school-segregation_n_860857.html).
- Lohman, Judith. 2010. The Racial Imbalance Law. OLR Research Report. Retrieved May 1, 2015 (http://www.cga.ct.gov/2010/rpt/2010-R-0228.htm)
- (Orfield, Gary and Ee, Jongyeon. 2015. Connecticut School Integration: Moving Forward as the Northeast Retreats. UCLA The Civil Rights Project 5. Retrieved April 29, 2015. (http://civilrightsproject.ucla.edu/research/k-12-education/integration-and-diversity/connecticut-school-integration-moving-forward-as-the-northeast-retreats/orfield-ee-connecticut-school-integration-2015.pdf)
- 2014. History of Sheff v. O’Neill. Sheff Movement: Quality Integrated Education for All Children. Retrieved May 1, 2015. (http://www.sheffmovement.org/history-2/)
- 2014. Sheff v. O’Neill. Wikipedia: The Free Encyclopedia. Retrieved May 1, 2015. (http://en.wikipedia.org/wiki/Sheff_v._O’Neill)
- Zirkel, Sabrina. 2004. What Will You Think of Me? Racial Integration, Peer Relationships and Achievement Among White Students and Students of Color. Mills College: Saybrook Graduate School and Research Center. Journal of Social Issues, Vol. 60, No. 1, 57-74
- (Spencer, Marguerite. 2009. The Benefits of Racial and Economic Integration in Our Education System:Why this Matters for Our Democracy. The Kirwin Institute. 1-19.)
- (Tegeler, Philip, Mickelson, Roslyn Arlin and Bottia, Martha. 2011. “Research Brief No. 4: What we know about school integration, college attendance, and the reduction of poverty.” Poverty and Race Research Action Council. The National Coalition on Student Diversity 1-4.”)
- Thomas, Jacqueline Rabe. 2014. 60 Years after Brown vs. Board of Education: Still Separate in Connecticut. The CT Mirror. Retrieved May 1, 2015. (http://ctmirror.org/2014/05/16/60-years-after-brown-vs-board-of-education-still-separate-in-connecticut/)
- 2015. Connecticut Magnet Schools. Public School Review. Retrieved May 11, 2015. (http://www.publicschoolreview.com/state_magnets/stateid/CT)
- Chen, Grace. 2015. What is a Magnet School? Public School Review. Retrieved May 3, 2015. (http://www.publicschoolreview.com/blog/what-is-a-magnet-school)
- 2013. What are Magnet Schools? Magnet Schools of America. Retrieved April 29, 2015. (http://www.magnet.edu/about/what-are-magnet-schools)
- Rossell, Christine. 2003. “The Desegregation Efficiency of Magnet Schools.” Boston University Political Science Department. Urban Affairs Review 1-25)
- Kahlenberg, Richard D and Potter, Haley. “Charter Schools” Can Racial and Socioeconomic Integration Promote Better Outcomes for Students? Poverty and Race Research Action, The Century Foundation.
- Beaudin, Barbara Q. Interdistrict Magnet Schools in Connecticut. Connecticut State Department of Education Division of Evaluation and Research. Retrieved May 2, 2015. (http://www.sde.ct.gov/sde/lib/sde/PDF/Equity/magnet/magnet_presentation_caims_11_2003.pdf)
- Thomas, Jacqueline Rabe. 2014. Report: Many Connecticut charter schools ‘hyper-segregated’. The CT Mirror. Retrieved May 2, 2015. (http://ctmirror.org/2014/04/09/school-choice-many-schools-hyper-segregated/)
- Orfield, Gary. 2015. Race and Schools: The Need for Action. National Education Association. Retrieved May 11, 2015. (https://www.nea.org/home/13054.htm_)
- (2015. School Desegregation and Equal Education Opportunity. The Leadership Conference Civil Rights 101. Retrieved May 11, 2015. (http://www.civilrights.org/resources/civilrights101/desegregation.html))
- Dreher, Rod. 2013. Can We Ever ‘Fix’ Segregated Schools? The American Conservative. Retrieved May 11, 2015. (http://www.theamericanconservative.com/dreher/can-we-ever-fix-segregated-schools/)
- Kahlenberg, Richard D and Potter, Haley. 2014. A Smarter Charter: Finding what Works for Charter Schools and Public Education. New York, NY: Teachers College Press
- Thomas, Jacqueline Rabe. 2015. School choice: Future of new magnet schools uncertain. The CT Mirror. Retrieved May 3, 2015. (http://ctmirror.org/2015/01/06/school-choice-future-of-new-magnet-schools-uncertain/)